- AiNews.com
- Posts
- Google’s AI Co-Scientist: How AI Is Accelerating Scientific Discovery
Google’s AI Co-Scientist: How AI Is Accelerating Scientific Discovery
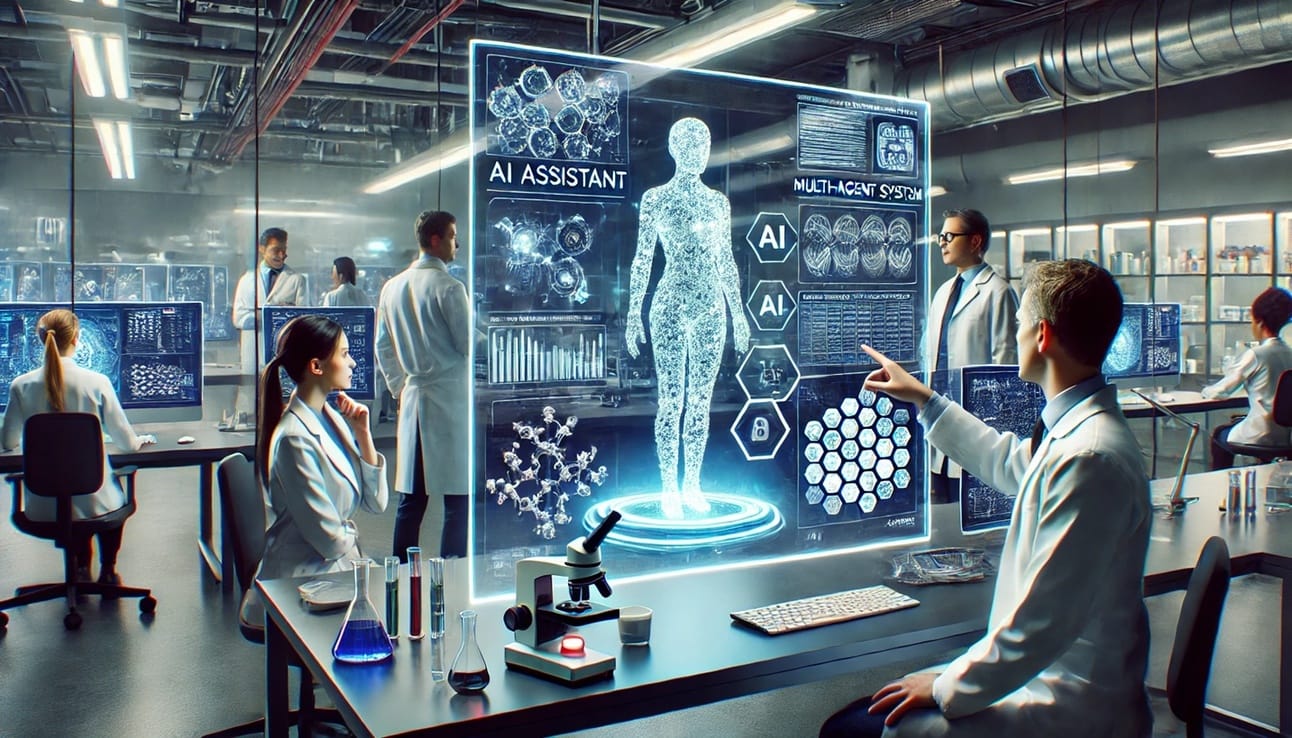
Image Source: ChatGPT-4o
Google’s AI Co-Scientist: How AI Is Accelerating Scientific Discovery
Google has unveiled an advanced AI co-scientist, a multi-agent AI system built on Gemini 2.0, designed to function as a virtual scientific collaborator. The system is intended to assist researchers in generating new hypotheses, formulating research proposals, and accelerating scientific and biomedical discoveries.
Addressing Challenges in Scientific Research
Scientific breakthroughs often require interdisciplinary expertise, but keeping up with the rapid expansion of scientific literature and integrating insights from multiple domains is challenging. Many of the most significant advances—such as Emmanuelle Charpentier and Jennifer Doudna’s 2020 Nobel Prize-winning work on CRISPR—have emerged from transdisciplinary research.
The AI co-scientist seeks to bridge this gap by leveraging AI-powered reasoning, long-term planning, and synthesis of complex subjects. Unlike standard literature review and summarization tools, it is designed to uncover original insights, propose new research directions, and formulate hypotheses based on prior evidence—all tailored to specific research goals.
How the AI Co-Scientist Works
The system operates through a coalition of specialized AI agents, each aligned with different aspects of the scientific method:
Supervisor – Oversees research plans, assigns tasks, and manages computational resources
Generation – Creates initial research hypotheses
Reflection – Analyzes and critiques hypotheses
Ranking – Prioritizes the most promising hypotheses
Evolution – Refines hypotheses for improved quality
Proximity – Ensures hypotheses align with existing evidence
Meta-review – Conducts final assessments
These agents work together in an iterative, self-improving cycle, using automated feedback to enhance the quality of outputs. Scientists can also interact with the system, providing feedback or guiding research directions. The Supervisor agent manages research plans, allocates computing resources, and scales processing power to improve reasoning. In addition to its specialized agents, the AI co-scientist leverages web search and specialized AI models to enhance the accuracy and relevance of its generated hypotheses, ensuring they are well-grounded in existing scientific knowledge.
Advancing AI-Driven Scientific Reasoning
The AI co-scientist employs test-time compute scaling to refine hypotheses through processes such as:
Self-play scientific debate – Generates and tests new ideas
Ranking tournaments – Compares hypotheses for quality
Evolution cycles – Enhances reasoning and self-improvement
The system's Elo auto-evaluation metric, adapted from ranking models, ensures higher-rated outputs correlate with more accurate and impactful discoveries. In a study of 15 open research challenges, the AI co-scientist outperformed other state-of-the-art models, demonstrating its ability to improve scientific reasoning with increased computing time. This performance was further validated by the GPQA benchmark, where higher Elo ratings correlated with greater accuracy on complex scientific questions.
Real-World Validation in Biomedical Research
To assess its practical impact, the AI co-scientist was tested in laboratory experiments across three key biomedical applications:
Drug Repurposing for Acute Myeloid Leukemia (AML)
AI-generated hypotheses identified potential new uses for existing drugs.
Clinician feedback and in vitro experiments confirmed that proposed drugs inhibited tumor viability at clinically relevant concentrations.
New Treatment Target Discovery for Liver Fibrosis
AI-assisted target discovery focused on epigenetic factors with significant anti-fibrotic activity.
Research findings will be detailed in an upcoming report from Stanford University.
Antimicrobial Resistance (AMR) Mechanisms
AI-generated hypotheses explored how capsid-forming phage-inducible chromosomal islands (cf-PICIs) expand bacterial host ranges. Through its analysis, the AI co-scientist independently proposed that cf-PICIs interact with diverse phage tails to expand their host range, offering new insights into bacterial gene transfer mechanisms.
These AI-driven insights were independently validated against pre-existing unpublished research from Imperial College London and the Fleming Initiative.
Solving a Superbug Mystery in Two Days
In a striking demonstration of its capabilities, the AI co-scientist solved a decade-long scientific mystery in just two days. Professor José R. Penadés and his team at Imperial College London had spent years investigating how some superbugs (antibiotic-resistant bacteria) develop and spread. When they tested Google's AI tool with a short prompt describing their research challenge, it generated the correct hypothesis within 48 hours—a conclusion that had taken years to reach through traditional methods.
Even more remarkably, the AI did not rely on publicly available data—the research had not been published or shared. The system also produced four additional hypotheses, all of which made scientific sense, including one that the research team had never considered and is now actively exploring.
This experiment underscores the potential of AI to radically accelerate scientific discovery, reducing research timelines from years to days while also generating novel ideas that human researchers might overlook.
Limitations and Future Potential
While promising, the AI co-scientist requires improvements in:
Enhanced literature reviews and fact-checking
Cross-validation with external tools
Expanded evaluation with more domain experts
Despite these challenges, the AI co-scientist represents a significant step toward AI-assisted scientific discovery. By autonomously generating testable hypotheses and refining its reasoning, the system has the potential to accelerate research in medicine and beyond.
Expanding Access Through a Trusted Tester Program
To further evaluate the AI co-scientist’s strengths and limitations, Google is launching a Trusted Tester Program, allowing research organizations to access and explore its capabilities. Interested institutions can apply to join the program.
What This Means
AI is playing an increasingly central role in scientific discovery and innovation. Google's AI co-scientist demonstrates how collaborative AI systems can support researchers by generating testable hypotheses, accelerating breakthroughs across disciplines, and streamlining complex research processes. By leveraging automated reasoning, external data sources, and self-improving feedback loops, AI-powered tools like this have the potential to significantly enhance scientific productivity and unlock discoveries that might otherwise take years to develop.
While human expertise remains essential, AI-driven approaches can augment scientists' capabilities, helping them navigate vast scientific literature, propose innovative solutions, and validate research directions more efficiently. As AI continues to evolve, its role in science and medicine will likely expand, providing researchers with powerful tools to tackle some of the world’s most pressing challenges.
The AI co-scientist is more than just a research assistant—it is a true collaborator, capable of dramatically shortening research timelines. The Imperial College London study is a striking example: in just two days, AI reached the same conclusion that took human scientists a decade to uncover. Even more remarkably, it generated four additional hypotheses, one of which researchers are now actively exploring.
Rather than replacing human scientists, AI acts as a force multiplier, allowing researchers to test ideas faster, uncover new possibilities, and focus on real-world validation. If AI can solve decade-old mysteries in days, the future of accelerated scientific breakthroughs is no longer theoretical—it is already happening.
Editor’s Note: This article was created by Alicia Shapiro, CMO of AiNews.com, with writing, image, and idea-generation support from ChatGPT, an AI assistant. However, the final perspective and editorial choices are solely Alicia Shapiro’s. Special thanks to ChatGPT for assistance with research and editorial support in crafting this article.